![📚 Python Lists Demystified: A Beginner's Guide for Social Research" [Part 2 of 3]](https://images.squarespace-cdn.com/content/v1/67338986b9d79a68ac92b01e/1735945779298-LJ9W1NZZX0B4WDGKQGDU/python+lists+2a.png)
📚 Python Lists Demystified: A Beginner's Guide for Social Research" [Part 2 of 3]
Learn Python lists for social research data analysis! This beginner-friendly guide shows how to use Python's built-in list functions (len(), count(), min(), max()) to analyze survey responses, manage participant data, and organize research results.
Perfect for social scientists transitioning to Python programming, this tutorial uses real research scenarios to demonstrate how to count responses, validate data, find patterns, and work with multiple datasets.
Part 2 of our 3-part series on Python for social research data analysis.
![📚 Python Lists Demystified: A Beginner's Guide for Social Research" [Part 1 of 3]](https://images.squarespace-cdn.com/content/v1/67338986b9d79a68ac92b01e/1734644941099-CJZBR6MPQ32DCGYJ4O09/picture-indiana-jones--but-instead-of-hunting-anci.jpg)
📚 Python Lists Demystified: A Beginner's Guide for Social Research" [Part 1 of 3]
Learn how to efficiently manage research data with Python lists!
This beginner-friendly guide shows social researchers how to organize, sort, and manipulate data collections using Python's built-in list methods. Master essential operations like sorting survey responses, reversing chronological data, and handling participant information.
Perfect for researchers transitioning from spreadsheets to Python, with practical examples and step-by-step instructions. No programming experience required.
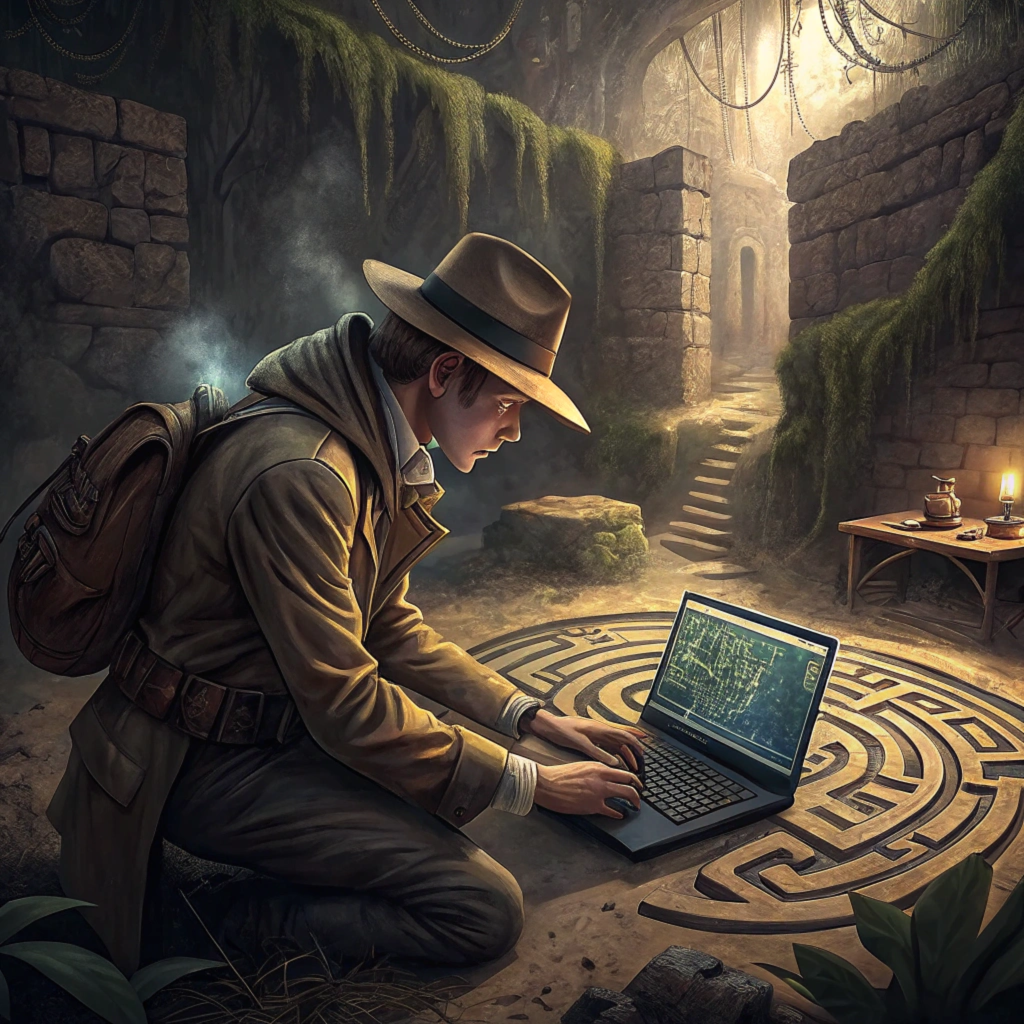
🐍 Built-in Python Functions: Essential Tools for Social Scientists
Learn how to automate your social science research with Python's 12 essential built-in functions. Transform hours of manual Excel work into minutes of efficient data processing.
Perfect for researchers and social scientists looking to streamline survey analysis, clean datasets, and generate reproducible results.
Discover how functions like map(), filter(), and zip() can revolutionize your data workflow—no advanced programming knowledge required. Includes practical examples from real social research scenarios and step-by-step tutorials.
![🧵 First Steps with Text Strings in Python: [Part 3 of 3]: Transforming Text with Essential Methods](https://images.squarespace-cdn.com/content/v1/67338986b9d79a68ac92b01e/1732742431739-JVA10ENC0MS4J2GE2CW9/python+methods+5.png)
🧵 First Steps with Text Strings in Python: [Part 3 of 3]: Transforming Text with Essential Methods
Learn essential Python string methods for data cleaning and text processing in qualitative research.
This practical guide shows you how to automate common tasks like standardizing survey responses, cleaning interview transcripts, and processing research codes.
Perfect for social science researchers and qualitative data analysts, this tutorial covers key string manipulation techniques including case standardization, whitespace removal, and pattern matching.
Through real-world examples and hands-on exercises, you'll master Python methods that can reduce hours of manual text processing to minutes. Whether you're handling interview transcripts, survey responses, or research codes, these string manipulation techniques will streamline your qualitative data analysis workflow.
![🧵 First Steps with Text Strings in Python: [Part 2 of 3]: f-strings, Indexing, and Slicing](https://images.squarespace-cdn.com/content/v1/67338986b9d79a68ac92b01e/1732652437876-QEJ7K85F08MDKBA4PV31/f-strings+3.png)
🧵 First Steps with Text Strings in Python: [Part 2 of 3]: f-strings, Indexing, and Slicing
Master essential Python string operations with this comprehensive guide for beginners and intermediate programmers. Learn how to use modern f-strings for efficient text formatting, understand string indexing for precise character access, and master string slicing for text manipulation. Perfect for data analysts, researchers, and developers working with text processing.
Key topics covered:
Modern string formatting with Python f-strings
String indexing for character-level manipulation
Text slicing techniques for data extraction
Practical examples with real-world applications
Hands-on challenges with detailed solutions
Whether you're analyzing survey responses, processing research data, or developing text-based applications, this tutorial provides the fundamental tools you need for effective string manipulation in Python 3.6+.